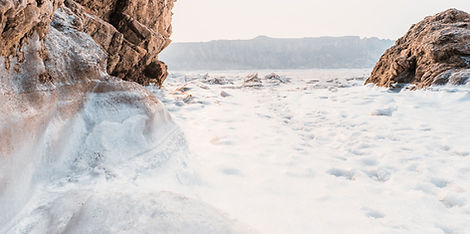
Doctoral Research Ideas
My Doctoral Research Interests Focus on How to Support Authentic Learning and Teaching in Mobile Technology-integrated Learning Environment.
The research ideas that guide my doctoral study and research are:
1. What are the effects of mobile learning on student learning and achievement?
2. What factors might impact the effects of mobile learning on student learning and achievement?
3. How to design mobile applications in term of advancing students cognition and computational thinking?
4. What are student's perception and emotion of using mobile technologies in learning.
5. What are the design principles of mobile learning-integrated learning environment?
During my first-year doctoral study, I did much research on investigating the effect of mobile learning on students science learning and achievement and examined the impact factors from the pedagogical perspective. In this regard, I have one mobile learning paper published and one mobile learning manuscript under preparation.
First, the paper Understanding How the Perceived Usefulness of Mobile Technology Impacts Physics Learning Achievement: a Pedagogical Perspective was published on Journal of Science Education and Technology.
In this study, we found that the relationship between the perceived usefulness and the use frequency of mobile technology varied significantly across the three pedagogical categories (i.e., student-led, teacher-led, and collaborative). We also found the use frequency mediated the relationship between perceived usefulness and physics learning achievement particularly for the collaborative functions. The male and female students tended to have different perceptional accountability of mobile technology for their physics learning achievement. Specifically, male students’ perception of collaborative functions positively predicted their physics learning achievement, while female students’ perception of student-led functions positively predicted their physics learning achievement. Both male and female students’ perception of teacher-led functions negatively predicted their physics learning achievement.
Second, I am working on a meta-analysis journal paper Examining how the pedagogy role moderates the mobile learning effect on student science achievement: A meta-analysis.
Research statement
Mobile technologies have significantly changed the science learning paradigm by extending the learning space and learning time and providing various mobile toolkits. Much research investigated the different pedagogy ways of mobile technologies in improving student learning achievement in science education. However, we do not have a comprehensive understanding of the ML pedagogy benefits across individual studies with research-based evidence. To fill this gap, we performed a meta-analysis of 58 effect sizes in 34 studies in science education to examine how students’ and teachers’ pedagogy roles varied, as well as how the variations moderated the ML effects on students’ science achievements. The research questions guiding this meta-analysis were:
(1) What is the heterogeneity and the weighted mean effect of ML on student science learning achievement?
(2) What does the pedagogy role moderate ML effect on student science learning achievement?
Three-dimensional framework
In science classrooms, it is hard to assume that mobile technology’s introductioin into science learning could automatically encourage students to construct their internal representations. In a recent study, Zhai, Li, and Chen (2019)identified “who leads the use” as a key mobile technology’s pedagogical and developed an STC three-dimensional model (i.e., student-led, teacher-led, and collaborative) (See Fig. 1). We adopted the STC model to analyze teachers and students pedagogy role.
Figure 1. The three-dimensional STC model, adopted from Zhai et al. (2019)
Based on constructivism in education (Staver, 1998), one given technology could have different approaches to be used in learning and teaching, which could be student-led or teacher-led using. The student-led pedagogy is students initiate, decide and lead the use of mobile technology regarding when and how to use it (Zhai & Shi, 2020). Teachers have no or minimum influence on students’ use of mobile devices. Teachers’ role shifted from “sage on the stage” to “guide on the side” (Johnson, 1995).
The teacher-led pedagogy indicates that teachers initiate, decide, and lead the use of mobile technology (Zhai & Shi, 2020). In this situation, students are usually passively influenced by mobile technology with no or minimum contribution to the use of it. At the same time, teachers decide when to use and how to use mobile technology with the main purpose of facilitating their teaching activities.
Collaborative pedagogy means both students and teachers play active roles in using mobile technology. The mobile technology use can be initiated by either the teacher or students and the counterpart has active input (Wette, 2015). This means both teachers and students are positively involved in deciding when and how to initiate mobile technology’s use and having their input.
Method
We followed the meta-analysis procedures suggested by Glass, Smith, and McGaw (1981). Literature from 2010 to 2020 was searched in five databases ( see Fig. 2). The initial search yielded 1975 articles. The combination of two sets of keywords (see Fig. 2) was used as filters to search in each database.
We specified a set of comprehensive inclusion and exclusion criteria to identify the eligible studies (Luaces, Díez, & Bahamonde, 2018).
1. Studies had to be peer-reviewed journal articles published between 2010 and 2020.
2. The application of mobile devices was the key variable of studies.
3. Studies that reported ESs directly or provided sufficient information to calculate ESs were included in this meta-analysis.
4. The student learning achievement as the major dependent variable had to be clearly described with quantitative data.
We retrieved 1975 studies and coded them that had to be experimental or quasi-experimental between-subject designs, and with sufficient information to calculate effect sizes. The interrater reliability of Cohen’s was 0.651, or medium agreement (McHugh, 2012; Nehm & Haertig, 2012). Finally, we included 34 studies with 59 independent effect sizes in this meta-analysis. An adapted PRISMA flow diagram (Moher, Liberati, Tetzlaff, Altman, & Group, 2009) for the number of studies identified, screened, ultimately eligible, and included can be viewed in Figure 2.
Figure 2. Flow chart of literature selection and coding process
We used the esc package in R to calculate the Hedge’s. The interpretative cutoff proposed by Cohen (1968)suggested an effect size of 0.20 is considered small, 0.50 is considered medium, and 0.80 is considered large. In this study, we will use this cutoff to report our results.
Results
Main effect and heterogeneity analysis
We used the metafor package in R to do the meta-analysis. This meta-analysis captured data from 5673 K-12 and university students across 34 studies , yielding 58 independent effect sizes. Quantifying heterogeneity is vital to choose the appropriate model (M. Borenstein, L. V. Hedges, J. P. Higgins, & H. R. Rothstein, 2011). If the effect sizes of all studies present a homogeneous distribution in the heterogeneity test result, we should adopt a fixed-effect model. Otherwise, a random-effect model is appropriate (Michael Borenstein et al., 2011). The heterogeneity test was significant, which indicated the heterogeneous distribution of effect sizes between studies. The I2 was calculated at 67.58%, which suggested the heterogeneity within the present study is moderate to substantial heterogeneity (Higgins, Thompson, Deeks, & Altman, 2003). The result indicated the random-effect model is appropriate.
The random-effect model showed the estimated Hedge’s was 1.04 (95% CI [0.82-1.27]), indicating a Substantial effect (Cohen, 1968) (See Fig 3). Z-score, indicating the difference in learning achievement between the experiment and control group, was statistically significant (Z = 9.07, p <.0001).
Figure 3. The forest plot of the main effect size
The moderator effect of pedagogical role
The mixed-effect mode with the moderator variable pedagogy role was used to examine whether and at what degree pedagogy role contributed to the significant heterogeneity of our samples. The fit statistics results showed that the pedagogy role could explain 33.32% of the unaccounted variability at a significant moderator effect with Qm = 87.98, df = 3, p<.0001, under significant level a = .05 .
The student-led and collaborative pedagogy roles were significant moderators at a significant level . The estimated Hedge’s for student-led was Substantial (𝑔 = 1.19, 95%CI = [0.91, 1.48]) (Cohen, 1968) with statistical significance (p <.0001 ). The collaborative was also a significant predictor with High ES (𝑔 = 0.97,95%CI = [0.48, 1.47] ) and ( p<.0001 ) However, the teacher-led moderation effect was not significant at p = 0.348 ), with a Moderate ES (𝑔 = 0.58, 95%CI = [0.06, 1.10]).
Discussion
This study extended our understanding of ML pedagoggical features through research-based evidence. On avearge, ML can improve students science achievement by 1.04 standard deviations. This indicated that ML intervention is significantly more effective when compared to traditional teaching without including ML. In addition, the random-effect model explained 67.58% variance of ML effect.
The pedagogical role of ML had a statistically significant moderator effect in student science achievement. Specifically, student-led pedagogy of ML had highest moderator effect, followed by collaborative and teacher-led pedagogical roles. The student-led pedagogy can improve student science achievement by 1.19 standard deviations, which was much higher than that from the mean effect. This result is most likely due to the fact that student-led pedagogy supports learner autonomy, which can improve student engagement and motivation in learning (S. W. Park, 2017). The effect size associated with collaborative uses of ML was also high and statistically significant. In this study, the collaborative pedagogy included the teacher, supporting studies that have found that students benefit from ongoing guidance and input from the teacher (Li & Wang, 2018; Y. Park, 2011; Zhai et al., 2019). The findings consistent with prior research suggested that instructional designers and science educators should consider mobile technology’s pedagogy feature when applying ML in science education.
Implications
The study suggests how instructional designers and science educators should consider ways to support student learning through student-led and collaborative pedagogy roles of ML. There are currently several ways of doing this in science education. Project-based learning (PBL) is a popular approach that supports student-led inquiry in solving real-world science problems. Mobile technology can play an important role in supporting PBL because it offers students access to various information as well as software tools that can support them in presenting their products (e.g., presentation, an artifact, data analysis) (Krajcik & Blumenfeld, 2006).
Third, how to design, develop, and implement the mobile application to support authentic learning and evaluate its effectiveness?
The innovation in science learning
The National Research Council Framework (NRC, 2012) and the Next Generation Science Standards ([NGSS]; NGSS Lead States, 2013) have set forth an ambitious vision of three-dimensional (3D) science learning (i.e., integrating science and engineering practices, disciplinary core ideas, and crosscutting concepts).
(2) The 3D science learning goals require the transformation of instruction and learning. It requires students to participate in scientific practices to develop knowledge-in-use (Delen & Krajcik, 2017).
The great potential of using mobile technologies in science learning
(1) Mobile devices expand the study of science beyond the traditional classroom walls due to its portability and mobility.
(2) Mobile devices provide multiple scientific toolkits with embedded cameras, video recorders, multimedia communication, sensors, and many smart functions (Sawyer, 2005) to support various learning activities in authentic learning settings.
The research gap of using mobile applications in learning
(1) Much research examined the effect of mobile application on students achievement and affective.
(2) Less research investigated how to develop students computational thinking through the use of mobile application. Also, not so much research examined the effect of mobile learning from the perspective of students' metacognition and emotion.
Reference
Azevedo, R., & Bernard, R. M. (1995). A meta-analysis of the effects of feedback in computer-based instruction. Journal of Educational Com- puting Research, 13(2), 111–127.
Bai, H. (2019). Pedagogical practices of mobile learning in K-12 and higher education settings. TechTrends, 63(5), 611-620.
Bano, M., Zowghi, D., Kearney, M., Schuck, S., & Aubusson, P. (2018). Mobile learning for science and mathematics school education: A systematic review of empirical evidence. Computers & Education, 121, 30-58
Beggrow, E. P., Ha, M., Nehm, R. H., Pearl, D., & Boone, W. J. (2014). Assessing scientific practices using machine-learning methods: How closely do they match clinical interview performance? Journal of Science Education and Technology, 23(1), 160–182
Borenstein, M., Hedges, L., Higgins, J., & Rothstein, H. (2011). Introduction to Meta-analysis. Wiley. com.
Cheung, W. S., & Hew, K. F. (2009). A review of research methodologies used in studies on mobile handheld devices in K-12 and higher education settings. Australasian Journal of Educational Technology, 25(2).
Cohen, J. (1960). A coefficient of agreement for nominal scales. Educational and psychological measurement, 20(1), 37-46.
Cohen, J. (1968). Multiple regression as a general data-analytic system. Psychological bulletin, 70(6p1), 426.
Crompton, H., Burke, D., & Lin, Y. C. (2019). Mobile learning and student cognition: A systematic review of PK‐12 research using Bloom’s Taxonomy. British Journal of Educational Technology, 50(2), 684- 701.
Duval, S., & Tweedie, R. (2000). A nonparametric “trim and fill” method of accounting for publication bias in meta-analysis. Journal of the american statistical association, 95(449), 89-98.
Fu, Q.-K., & Hwang, G.-J. (2018). Trends in mobile technology-supported collaborative learning: a systematic review of journal publications from 2007 to 2016. Computers & Education, 119, 129-143.
Glass, G. V., Smith, M. L., & McGaw, B. (1981). Meta-analysis in social research: Sage Publication, Incorporated.
Higgins, J. P., Thompson, S. G., Deeks, J. J., & Altman, D. G. (2003). Measuring inconsistency in meta-analyses. Bmj, 327(7414), 557-560.
Jescovitch, L. N., Scott, E. E., Cerchiara, J. A., Merrill, J., Urban-Lurain, M., Doherty, J. H., & Haudek, K. C. (2020). Comparison of machine learning performance using analytic and holistic coding approaches across constructed response assessments aligned to a science learning progression. Journal of Science Education and Technology, 1–18
Johnson, G. R. (1995). First steps to excellence in college teaching: ERIC.
Liu, O. L., Rios, J. A., Heilman, M., Gerard, L., & Linn, M. C. (2016). Validation of automated scoring of science assessments. Journal of Research in Science Teaching, 53(2), 215–233
Liu, C., Zowghi, D., Kearney, M., & Bano, M. (2021). Inquiry‐based mobile learning in secondary school science education: A systematic review. Journal of computer assisted learning, 37(1), 1-23.
Luaces, O., Díez, J., & Bahamonde, A. (2018). A peer assessment method to provide feedback, consistent grading and reduce students' burden in massive teaching settings. Computers & Education, 126, 283-295.
Martin, T., & Sherin, B. (2013). Learning analytics and computational techniques for detecting and evaluating patterns in learning: an introduction to the special issue. Journal of the Learning Sciences, 22(4), 511–520.
McHugh, M. L. (2012). Interrater reliability: the kappa statistic. Biochemia medica, 22(3), 276-282.
Moher, D., Liberati, A., Tetzlaff, J., Altman, D. G., & Group, P. (2009). Preferred reporting items for systematic reviews and meta-analyses: the PRISMA statement. PLoS med, 6(7), e1000097.
Nehm, R. H., & Haertig, H. (2012). Human vs. computer diagnosis of students’ natural selection knowledge: testing the efficacy of text analytic software. Journal of Science Education and Technology, 21(1), 56-73.
Park, S. W. (2017). Motivation Theories and Instructional Design. Foundations of Learning and Instructional Design Technology.
Pellegrino, J. W., Wilson, M. R., Koenig, J. A., & Beatty, A. S. (2014). Developing assessments for the Next Generation Science Standards: ERIC
Pollara, P., & Broussard, K. K. (2011). Student perceptions of mobile learning: A review of current research, Paper presented at the Society for information technology & teacher education international conference.
Prevost, L. B., Smith, M. K., & Knight, J. K. (2016). Using student writing and lexical analysis to reveal student thinking about the role of stop codons in the central dogma. CBE—Life Sciences Education, 15(4).
Rosenthal, R. (1979). The file drawer problem and tolerance for null results. Psychological bulletin, 86(3), 638.
Staver, J. R. (1998). Constructivism: Sound theory for explicating the practice of science and science teaching. Journal of Research in Science Teaching: The Official Journal of the National Association for Research in Science Teaching, 35(5), 501-520.
Suh, S. M. A. (2014). Mobile technology and applications for enhancing achievement in k-12 science classrooms: A literature review.
Sung, Y.-T., Chang, K.-E., & Liu, T.-C. (2016). The effects of integrating mobile devices with teaching and learning on students' learning performance: A meta-analysis and research synthesis. Computers & Education, 94, 252-275.
Talan, T. (2020). The Effect of Mobile Learning on Learning Performance: A Meta-Analysis Study. Educational Sciences: Theory and Practice, 20(1), 79-103.
Wette, R. (2015). Teacher-led collaborative modelling in academic L2 writing courses. Elt Journal, 69(1), 71- 80.
Zhai, X., Li, M., & Chen, S. (2019). Examining the uses of student-led, teacher-led, and collaborative functions of mobile technology and their impacts on physics achievement and interest. Journal of Science education and Technology, 28(4), 310-320.
Zhai, X., & Shi, L. (2020). Understanding How the Perceived Usefulness of Mobile Technology Impacts Physics Learning Achievement: a Pedagogical Perspective. Journal of Science education and Technology, 1-15.
Zhai, X., Shi, L., & Nehm, R. H. (2020). A Meta-Analysis of Machine Learning-Based Science Assessments: Factors Impacting Machine-Human Score Agreements. Journal of Science Education and Technology, 1-19.
Zydney, J. M., & Warner, Z. (2016). Mobile apps for science learning: Review of research. Computers & Education, 94, 1-17.
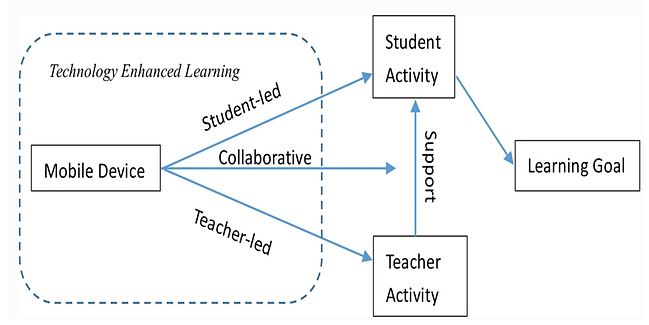
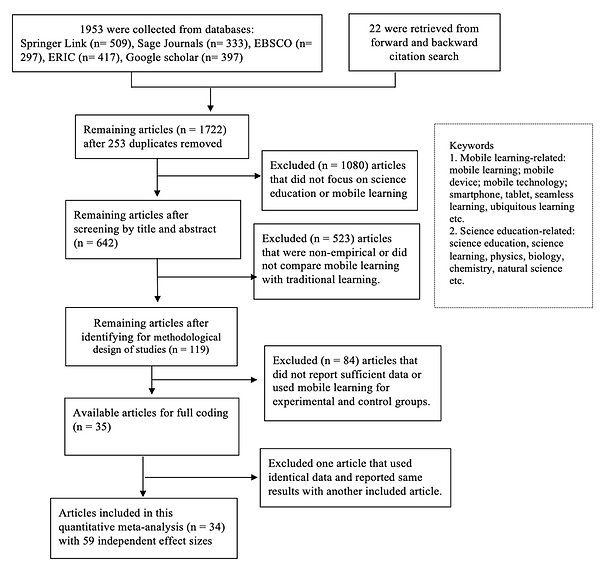
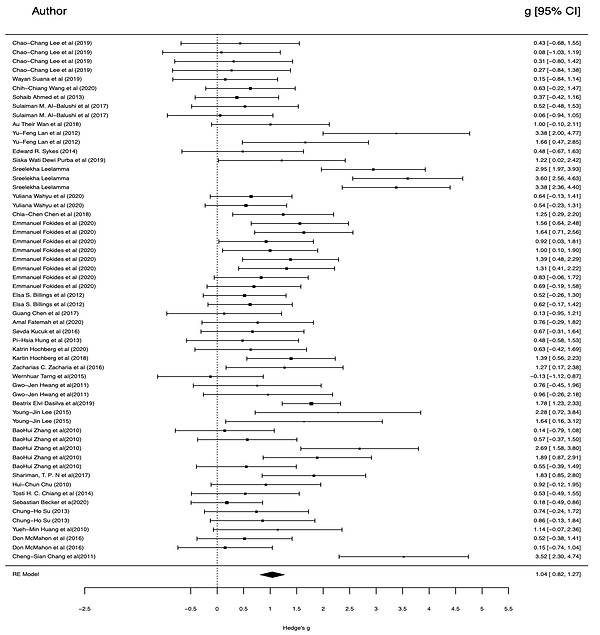